- Home
- Agencies
- Department of Agriculture
- Department of Housing and Urban Development
- General Services Administration
- Department of Commerce
- Department of the Interior
- National Aeronautics and Space Administration
- Department of Defense
- Department of Justice
- National Science Foundation
- Department of Education
- Department of Labor
- Office of Personnel Management
- Department of Energy
- Department of State
- Small Business Administration
- Environmental Protection Agency
- Department of Transportation
- Social Security Administration
- Department of Health and Human Services
- Department of the Treasury
- U.S. Agency for International Development
- Department of Homeland Security
- Department of Veterans Affairs
- Goals
- Initiatives
- Programs
Increase data scientists and data infrastructure
What is the Issue?
In December 2010, the President’s Council of Advisors on Science and Technology (PCAST) published a report to the President and Congress entitled: Designing a Digital Future: Federally Funded Research and Development in Networking and Information Technology.[1] In that report, PCAST pointed to the research challenges involved in large-scale data management and analysis and the critical role of Networking and Information Technology (NIT) in moving from data to knowledge to action, underpinning the Nation’s future prosperity, health and security.
Through long-term, sustained investments in foundational computing, communications and computational research, and the development and deployment of large-scale facilities and cyberinfrastructure, federal agency R&D investments over the past several decades have both helped generate this explosion of data and advanced our ability to capture, store, analyze, and use these data for societal benefit. More specifically, we have seen fundamental advances in machine learning, knowledge representation, natural language processing, information retrieval and integration, network analytics, computer vision, and data visualization, which together have enabled Big Data applications and systems that have the potential to transform all aspects of our lives.
These investments have produced tangible results, demonstrating the power of Big Data approaches across science, engineering, medicine, commerce, education, and national security, and laying the foundation for U.S. competitiveness for many decades to come. But much more needs to be done, particularly in four areas: 1) basic research; 2) data infrastructure; 3) education and workforce development; and 4) community outreach. In 2014 NSF endeavored to catalyze progress in these areas by developing programs to engage the research community, and by creating mechanisms to accelerate the development of people and infrastructure to address the challenges posed by this new flood of data.
What was the Intervention?
In FY 2014, NSF defined an Agency Priority Goal (APG) aimed at increasing the number of data scientists engaged in academic research, development, and implementation. As defined in the 2005 National Science Board (NSB) publication of Long-lived Digital Data Collections: Enabling Research and Education in the 21st Century defines data scientists as “the information and computer scientists, database and software programmers, disciplinary experts, curators, and expert annotators, librarians, archivists and others, who are crucial to the successful management of a digital data collection.”
Using its ability to convene diverse sets of stakeholders, NSF promoted multi-stakeholder partnerships by supporting workshops and follow-on activities that brought together representatives of industry, academia, not-for-profit organizations, and other entities to address current and future big-data challenges. NSF also leveraged existing programs, such as the NSF Research Traineeship (NRT) and the Graduate Research Fellowship (GRF) programs, and created new programs and tracks to current programs, as needed, to support the creation of more researchers and students competent in the deep analytical and technical skills required to address those challenges.
How was performance management useful?
Defining this effort as an APG focused agency-wide attention on implementing mechanisms to support the training and workforce development of future data scientists, increasing investments in current and future data infrastructure extending data-intensive science into more research communities, and increasing the number of multi-stakeholder partnerships to address the nation’s Big Data Challenges.
Led by Suzi Iacono, Office Head, Integrative Activities, and Joan Ferrini-Mundy, Assistant Director, Directorate for Education and Human Resources, a cross-agency team of eight met biweekly for two years to plan and implement this ambitious APG. The team recognized that the added structure of the goal pushed them to accomplish milestones more quickly than would have been possible if the activity had not been defined as an APG and acknowledged the positive and negative consequences of moving quickly. The leaders reported on their progress at quarterly data-driven performance reviews. Their accomplishments inspired other senior leaders who participated in these reviews to consider leading new APGs.
What were the outcomes and the impact?
Toward the aim of supporting the training and workforce development of future data scientists, NSF successfully inserted language emphasizing the education and training of data scientists in 18 solicitations, funded workshops for the community including: NAS Workshop: Training Students to Extract Value from Big Data, April 2014[1]; Advancing Data-Intensive Research in Education, June 2015[2]; and Graduate Data Science Workshop, August 2015[3] ; and added a Data-Enabled Science and Engineering (DESE) focus to the National Research Traineeship (NRT) program. The number of students in data science fields supported by GRF and NRT over the last three years is shown in Figure 1.
From FY13-FY15, NSF tracked a more than 25% increase in the number of degree and concentration, and certificate programs in data science at US universities.
Toward the aim of developing partnerships, NSF funded Four Big Data Innovation Hubs in FY 2015 to support partnerships that strive to achieve common big data goals that would not be possible to achieve alone.
Toward the aim of increasing investment in infrastructure, NSF issued new solicitations for funding opportunities (Building Community and Capacity in Data Intensive Research in Education (BCC), Data Infrastructure Building Blocks (DIBBS), and BIGDATA) and launched a challenge Prize to increase awareness of data science. To measure the number of communities/organizations/ecosystems that use data infrastructure and tools for their research and development (R&D) activities, NSF determined data intensiveness of NSF communities by monitoring the use of data-intensive high performance computing resources though Extreme Science and Engineering Discovery Environment (XSEDE). Compared to FY 2013, FY 2015 usage of XSEDE’s data intensive resources rose by 30 percent. The number of scientific disciplines using XSEDE rose by 25% (from 28 to 35 disciplines).
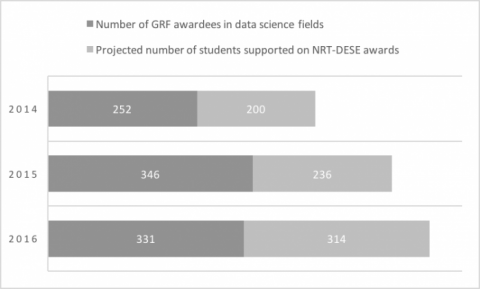